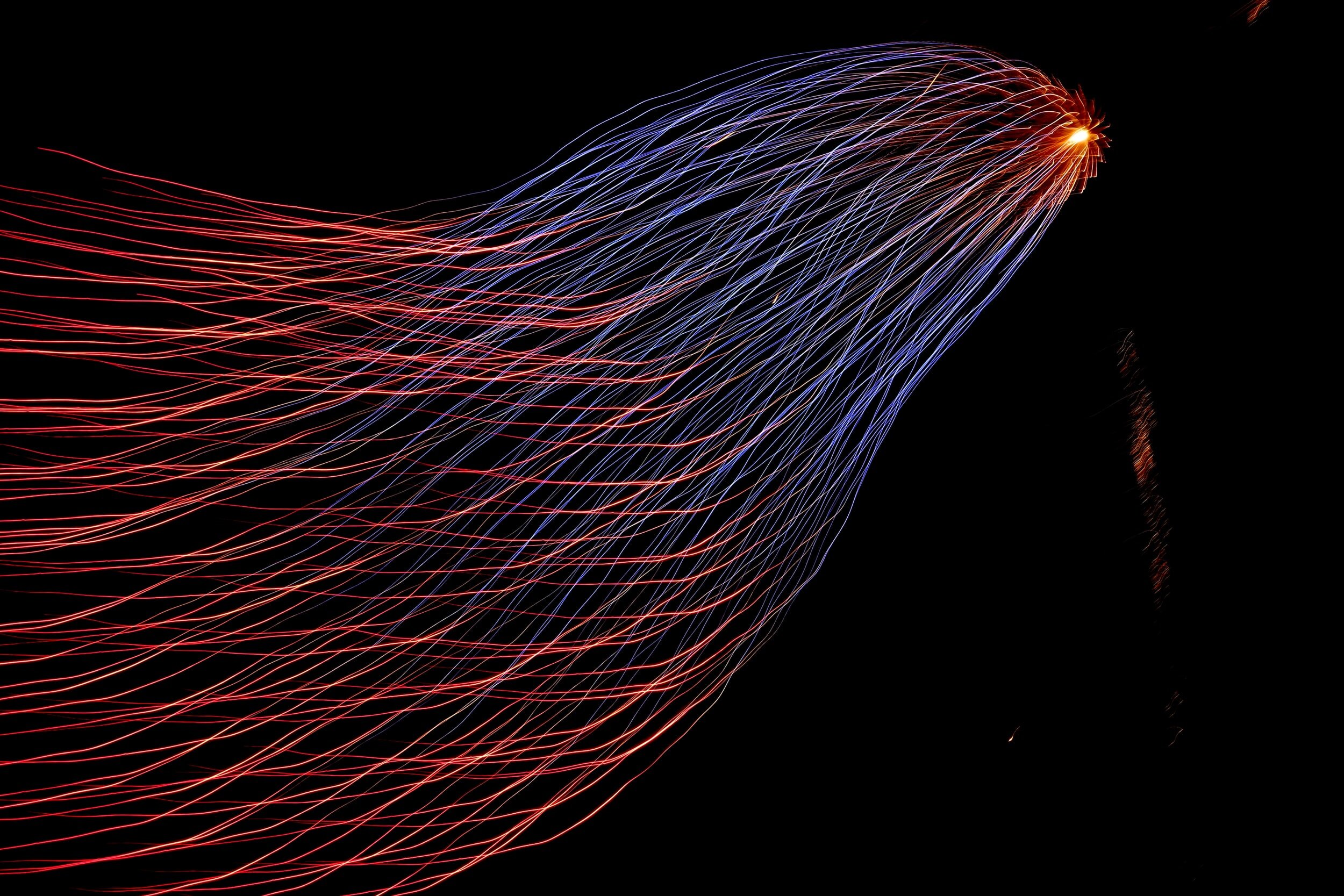
STREAMLINING MACHINE LEARNING MODEL DEPLOYMENT USING MLOPS
The Problem
A Fortune 500 client had recently formed a new machine learning team to analyze data for their business operations. However, without a proper pipeline and operation in place, the machine learning team quickly ran into deployment issues with different versions of Python and dependencies required for different projects. Without any standards in place, various model teams built their deployment tool sets by pulling in technologies like dockers and puppets. The homegrown tool sets were also difficult to maintain and not very modeler-friendly.
The Initiative
The client and GoodLabs decided to leverage a modified version of our GoodLabs MLOps framework for their machine learning team. This new MLOps practice was designed to standardize the deployment of their machine learning model as a function without any versions or dependency conflicts.
The Solution
We worked together with the Fortune 500 client to first identify their machine learning development process.
The machine learning team used Python as the only language in their model development. The issue with using Python is the version of Python required for different models due to the different dependencies. To worsen the matter, Python versioning is also part of the core requirement of the operating system. It is impossible to house many different versions of Python in a single system.
After many rounds of discussion with the Fortune 500 client’s machine learning team, we decided to use OpenFaaS to deploy each model in a separate container as a serverless function. OpenFaas allows each model to use different versions of Python with different versions of dependencies, without affecting other models or the operating system.
Additionally, all the models provide a calculation function for the business units and it does not require any state in place. Therefore, it is a perfect candidate to deploy as a serverless function.
The Result
When the new MLOps focused on using a standardized OpenFaaS framework to deploy machine learning models, this Fortune 500 client’s machine learning team was able to focus on their model training without diverting focus on building tool sets for deployment. Additionally, models no longer cause any version conflict with each other and the function can automatically scale up and down through OpenFaaS. The Fortune 500 client further reported their machine learning team having increased productivity by 65%.